AI in Science: Opportunities, Risks, and Strategies for the Future
- Andrea Viliotti
- 26 nov 2024
- Tempo di lettura: 11 min
Artificial intelligence (AI) is paving the way for a new golden age in science, as outlined in the document "A New Golden Age of Discovery: Seizing the AI for Science Opportunity" by Conor Griffin, Don Wallace, Juan Mateos-Garcia, Hanna Schieve, and Pushmeet Kohli. This document explores how AI can transform scientific disciplines, from genomics to materials science, and how it can be harnessed to address challenges of complexity and scale in research projects.
AI in science is transforming research and innovation in fields like genomics and materials. It accelerates experiments and modeling, as seen with AlphaFold, but demands ethical and sustainable strategies to balance risks and benefits. Investments in infrastructure, skills, and public-private collaboration are critical to expand global access, positioning AI as a catalyst for discoveries and applications.
Currently, AI is being used in laboratories worldwide to accelerate understanding, improve experimental precision, and generate new hypotheses. An example is AlphaFold, which provides protein structure predictions, drastically reducing research timelines that previously required years of work and resources. However, as this transformation continues to expand, it becomes crucial to understand how we can best leverage these new possibilities without ignoring the associated risks and responsibilities. How can we, therefore, balance the benefits with the potential risks, ensuring a safe and ethical use of AI in science?
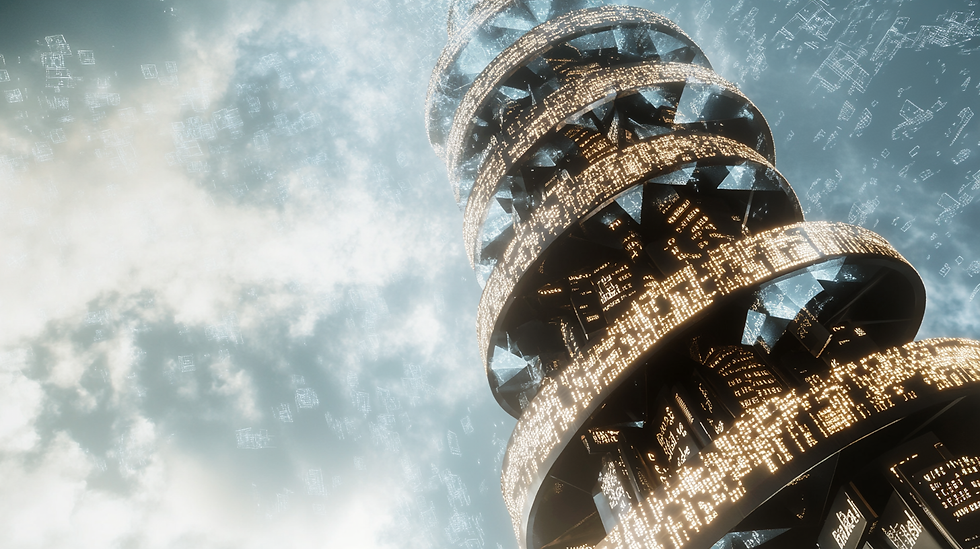
The Drive Behind AI Adoption in Science
In recent years, the growing interest in AI in science has been driven by a combination of social and technological pressures. Although the number of scientists and researchers has significantly increased, the pace of scientific discoveries has not kept up. This phenomenon is partly due to the greater complexity of problems being addressed today, as well as the need to assimilate an increasingly vast amount of existing knowledge. This growing knowledge burden requires more and more researchers to make new discoveries, making AI a valuable tool for overcoming limitations of scale and complexity.
One of the main factors driving AI adoption is its ability to accelerate processes that previously required enormous resources and time. For instance, while determining a protein structure through X-ray crystallography could take years of work and significant financial costs, the AlphaFold database now provides immediate access to 200 million predicted protein structures, helping to drastically reduce research time and costs.
AI is also transforming how science is practiced and shared. Today, one in three scientists uses large language models (LLMs) to support literature review, code writing, and document editing. This trend suggests a substantial shift in research activities, where AI is no longer just a computational tool but a true scientific assistant that supports the creation and communication of knowledge.
The adoption of AI in science is also seen as a necessary response to slowdowns in the growth of scientific productivity and progress towards global sustainable development goals. Recent decades have seen an acceleration in the creation of scientific knowledge, but also increasing difficulty in turning that knowledge into practical applications for society. Deep learning methodologies and advanced AI models can compress the time needed to achieve new advances, accelerating not only discovery but also the application of results in fields such as medicine, renewable energy, and materials science.
AI is therefore well-positioned to address problems of scale and complexity, helping to reduce the time and effort required to turn scientific discoveries into practical solutions. However, to fully realize AI's potential in science, a coordinated strategy is needed that includes investment in infrastructure, skills, and partnerships between the public and private sectors. Without a clear strategy, there is a risk that AI adoption will happen in a fragmented and ineffective manner, limiting the benefits it could offer to science and society.
Five Opportunities to Harness AI in Science
In many scientific disciplines, from computer science to structural biology, AI is opening new possibilities for discovery and innovation. Here are five key areas where AI can make a difference:
Knowledge: AI is transforming how scientists assimilate and communicate knowledge. The use of large language models allows rapid synthesis of information from an enormous number of academic publications, solving the problem of increasing specialization and the growing volume of existing knowledge. Recently, models like Gemini LLM have been used to extract relevant data from over 200,000 articles in a single day, enabling a much faster and more effective understanding of existing scientific literature. In a context where research is increasingly shared through preprints and code repositories, AI can also facilitate accessibility to this knowledge, adapting it for different audiences and making science more inclusive.
Data: Despite talk of a "data era," there are still enormous gaps in scientific information, especially in the natural sciences. AI can facilitate the collection, annotation, and cataloging of data, and even generate synthetic data to improve research. For example, AlphaProteo was developed using more than 100 million protein structures generated by AlphaFold, which were further enriched with experimental data from the Protein Data Bank. AI not only helps gather new data but can also leverage its ability to interpret unstructured data, such as images and audio, making available information that would otherwise be difficult to extract.
Experiments: Many scientific experiments are expensive and complex, and often cannot be conducted due to a lack of adequate resources. AI can simulate these experiments, reducing time and costs and optimizing the use of experimental resources. In nuclear fusion, for example, reinforcement learning agents have been used to simulate plasma control in a tokamak reactor, improving experimental efficiency. Similar techniques could also be extended to other large experiments, such as those conducted with particle accelerators or telescopes. This approach not only speeds up research but also helps more effectively identify optimal parameters for future experiments, avoiding costly mistakes and minimizing resource use.
Models: AIs can model complex systems and their interactions in ways that traditional deterministic models cannot. For example, weather systems are extremely dynamic and require high-resolution simulations to be accurately predicted. Deep learning models have been shown to predict weather conditions up to 10 days in advance, surpassing traditional models in terms of computation speed and forecast accuracy. This modeling capability can also be applied to economics, biology, and other fields where complex, interactive systems are the norm. Additionally, generative agent-based approaches allow scientists to create more flexible simulations that can respond and adapt to new conditions in real-time, such as simulating economic interactions between companies and consumers.
Solutions: Many scientific problems require exploring a practically infinite number of possible solutions, such as designing new drugs or materials. AI can explore these solution spaces more quickly and efficiently than traditional techniques based on intuition or empirical methods. For instance, AI models like AlphaProof and AlphaGeometry 2 have been able to solve complex mathematical problems, generating solutions that proved to be among the most accurate in international competitions. In biology, molecule design requires analyzing vast solution spaces, but AI can navigate these, quickly identifying the most promising options to test experimentally, as in the case of Covid-19 drugs and new classes of antibiotics.
The Risks of AI in Science
The adoption of artificial intelligence in science, while bringing immense opportunities, also raises several significant risks that require careful consideration. Among the main concerns are the negative impact on scientific creativity, the reduction of research reliability, the risk of diminishing theoretical understanding, the potential amplification of inequalities, and the environmental consequences related to the massive use of computational resources.
One of the primary risks is the potential reduction of scientific creativity. AI, particularly deep learning models, tends to emphasize regularity and minimize anomalies, while scientific creativity often arises from exploring those very anomalies. Many significant discoveries have resulted from unexpected observations and original insights. Relying solely on models that generalize from large amounts of data could lead to excessive standardization of the scientific process, reducing the potential to explore new and unusual paths. Moreover, the massive use of AI by different research groups could lead to homogenization of results, especially if the same models or datasets are used.
Another issue concerns the reduction of scientific reliability. Artificial intelligence, particularly large language models (LLMs), has shown a tendency to produce inaccurate or completely erroneous content, including fabricated citations. This phenomenon, known as "hallucination," poses a danger to science, where verification and replicability of results are fundamental. Furthermore, the use of AI in drafting scientific articles could encourage the proliferation of low-quality works, making it even more difficult to distinguish between reliable and unreliable information. This risk adds to those already present in the scientific community, such as publication bias and "p-hacking," which often lead to underestimating negative results.
An equally critical aspect concerns scientific understanding. Although AI can provide extremely accurate predictions, it often does not contribute to developing new theories or understanding phenomena. Science is not limited to predicting what will happen but aims to understand the "why." Current AI models, which rely on identifying patterns in data, risk turning science into a predominantly empirical activity, lacking the theoretical depth needed to understand underlying mechanisms. Without an adequate theoretical framework, AI predictions remain, in many cases, "black boxes," limiting scientists' ability to derive general insights applicable to new scenarios.
In terms of equity, the use of AI could exacerbate existing inequalities within the scientific community and between different parts of the world. Advanced AI technologies are mainly accessible to researchers in countries and institutions with ample financial resources, creating a barrier for those without access to such tools. This situation could widen the gap between well-funded institutions and those with fewer resources, limiting the participation of researchers from emerging economies and the diversity of voices in scientific research. Furthermore, the datasets used to train AI models often do not adequately represent diverse world populations, leading to less accurate results for underrepresented groups.
Finally, there are environmental risks associated with the use of AI in science. Training large models requires significant computational resources, resulting in energy consumption and increased greenhouse gas emissions. Although data centers represent only a fraction of global emissions, the growth in model sizes and their increasing adoption could significantly increase this impact. On the other hand, initiatives exist to make models more energy-efficient, and AI itself could be used to develop technologies aimed at reducing environmental impact, such as new materials for renewable energy or algorithms to optimize energy distribution.
To mitigate these risks, it is essential to adopt a strategy that includes responsible regulation of AI use, support for diversity in the scientific community, and the development of tools that make models more transparent and understandable. Moreover, policies should be promoted to ensure equitable access to AI technologies and encourage the sustainable use of computational resources, ensuring that scientific progress powered by AI can be shared equitably by all humanity.
AI and Global Innovation - Regulations and Strategies for Science
To fully exploit AI's potential in science, a clear and ambitious political strategy is needed at multiple levels. A fundamental first step is defining concrete scientific goals to guide AI research and use towards critical problems. The so-called "Hilbert Problems" for AI in science could provide an important platform for identifying the most pressing questions that AI could help solve. Governments and research organizations should launch initiatives to identify these problems, set clear parameters, and fund specific competitions that encourage scientists and engineers to find innovative solutions through AI. This approach would not only help concentrate resources and expertise on high-impact challenges but also provide a common vision shared at the international level.
An international network of Data Observatories for Science should be established to address chronic gaps in available scientific datasets, especially in underrepresented fields such as ecology, biodiversity, and social sciences. The observatories could conduct periodic "rapid data assessments" across various application fields, mapping existing gaps and identifying underutilized or hard-to-access datasets. These observatories could also promote the creation of new datasets that, if properly managed and maintained, could prove crucial for scientific progress. Such efforts must be supported by appropriate incentives for both individual researchers and institutions to ensure the sustainability and constant updating of data resources. It is crucial that data generated from strategic experiments are preserved and made accessible wherever possible, creating appropriate infrastructures for data storage and retrieval.
Another crucial aspect concerns the need to invest in training and skills development programs. AI is becoming an essential scientific tool and, as such, must become part of the educational curriculum for scientists at all levels. A wide range of training programs should be available, from introductory AI courses for undergraduates to specialized courses and fellowships for senior researchers. Moreover, every scientist should be able to access basic skills in using AI models to support their research, with courses covering responsible use of LLMs and model fine-tuning for specific research objectives. Only through extensive and deep scientific literacy on AI will it be possible to fully exploit AI's potential in research.
Computational infrastructure plays a determining role. Currently, many scientific institutions, particularly in low- and middle-income countries, do not have access to adequate computational resources. Governments must therefore fund shared infrastructures, such as public clouds dedicated to scientific research, to ensure all researchers have equitable access to the necessary computing power. At the same time, attention must be paid to the energy efficiency of these infrastructures to minimize their environmental impact. A sustainable approach to AI in science must include solutions that allow for the optimization of energy resource use through a combination of technological innovations and environmentally conscious infrastructural choices.
Public-private partnerships are essential for AI development in science. Collaborations with technology companies can accelerate the transfer of advanced technologies from research labs to practical applications. However, it is crucial that these collaborations are structured to ensure equitable access and that the benefits of innovation are shared with the community. Incentive policies such as tax breaks or funding for collaborative projects can stimulate cooperation between sectors, ensuring that the results of research born from these partnerships are in the public domain and available to the global scientific community.
Finally, an appropriate regulatory framework is needed to address the risks associated with AI use, such as model transparency, privacy protection, and security. Regulation should include guidelines to ensure AI models undergo rigorous verification and validation processes and that the data used for their training are managed ethically and responsibly. Promoting a culture of responsibility within the scientific community is also crucial, where researchers are aware of the ethical implications of their work and collaborate with policymakers and stakeholders to develop solutions that are safe, reliable, and respectful of human rights.
The adoption of AI in science is not a linear process and requires continuous adaptation. It will be essential to find a balance between human creativity and automation, between intuition and computational rigor. However, with appropriate policies and responsible use of AI, we could be at the beginning of a new period of discoveries that will make science more efficient, accessible, and capable of addressing the greatest challenges of our time.
Conclusions
The adoption of artificial intelligence (AI) in science is charting a path that offers crucial insights for the business world as well. AI's ability to transform complex processes into efficient and scalable solutions is a paradigm that companies must embrace not only as a technical tool but as a strategy for systemic innovation. However, the real challenge is not just technological but cultural: the way companies integrate AI will determine their ability to compete in an increasingly interconnected and knowledge-based market.
A fundamental first lesson is the need to balance speed and depth. In science, AI accelerates data collection and processing but must be anchored to strategic goals to avoid superficial or non-replicable results. Similarly, companies must avoid the "novelty syndrome"—the impulsive adoption of AI tools for marketing or trend-following reasons—and focus on implementations that have a tangible impact on core business. A key example could be the use of predictive models not only for market analysis but to anticipate structural trends, such as emerging consumer needs or supply chain vulnerabilities.
A second crucial aspect concerns the democratization of access to skills. Just as it is necessary in science to invest in training to make AI accessible to all researchers, in companies, it is essential to create an ecosystem where AI knowledge is not the exclusive domain of technical experts. AI literacy must extend to executives, marketing teams, and even functions traditionally distant from technology, such as human resources. This democratization not only fosters faster adoption but allows AI to generate value across the board.
However, the true competitive advantage emerges from the approach to collaboration. The public-private partnerships accelerating scientific innovation provide a replicable model for companies. Businesses must learn to work not only with their traditional stakeholders but also with external ecosystems, such as startups, universities, and research centers, to co-create AI-based solutions. Partnerships must be designed to share benefits and knowledge, avoiding situations where imbalances in technological or economic resources become obstacles to widespread innovation.
But the value of AI does not lie solely in its ability to produce efficiency; it lies in its potential to challenge the status quo. An important lesson from AI's use in science is the risk of flattening creativity and intuition, elements that remain central to both scientific discoveries and companies' competitive advantage. Companies should therefore view AI not as a replacement for human creativity but as an accelerator. An example is using AI to generate future scenarios that creative teams can explore, turning them into innovative and disruptive strategies.
Finally, the ethical and sustainable aspect of AI is a dimension that companies cannot overlook. Just as science must address the dilemma of energy consumption and AI model transparency, businesses must anticipate growing demands for accountability from consumers and regulators. Adopting sustainable, transparent, and inclusive AI practices will become not only a moral obligation but a differentiating competitive advantage, positioning companies as market leaders.
In summary, AI offers businesses an unprecedented opportunity to reimagine the future. However, success requires strategic vision, collaboration capacity, and an approach that balances technological innovation with human sensitivity.
Comments