Generative AI: Strategic Perspectives for Neuroscience, Security, and Governance
- Andrea Viliotti
- 26 dic 2024
- Tempo di lettura: 4 min
Aggiornamento: 2 gen
The growing complexity of the corporate and innovation landscape today arises from the convergence of multiple factors: from language models that surpass the expertise of human specialists to the integration of Generative AI into highly regulated industries such as banking, encompassing the need for specialized skills in the public sector, new security challenges related to LLM applications, increasingly complex game-based testing environments, and the establishment of control standards to avoid critical vulnerabilities. This is not merely a technological issue: it marks the advent of a scenario in which the ability to process, analyze, manage, and control AI becomes a true competitive, strategic, and cultural lever
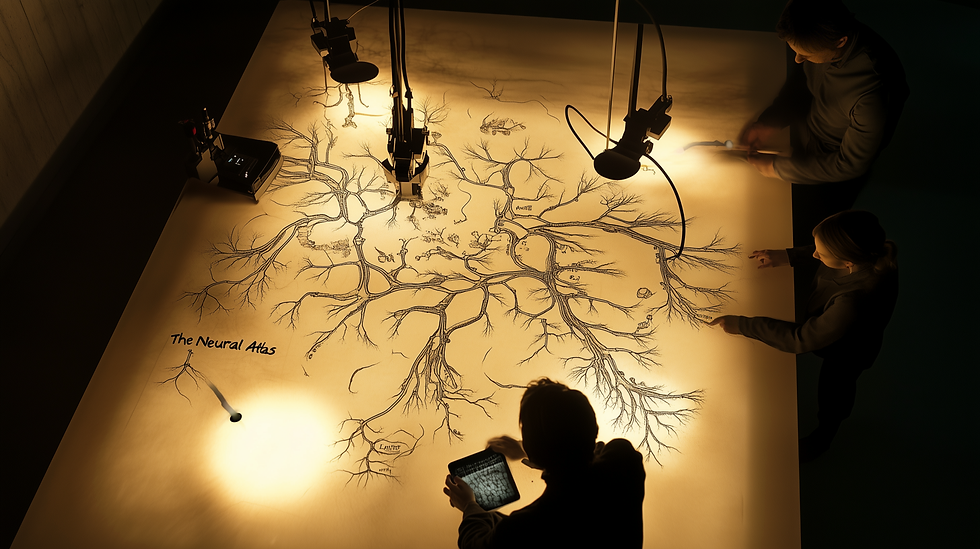
The complexity of LLMs comes into sharp focus when they are compared with areas of knowledge that were once the exclusive domain of high-level specialists. The article "BrainBench: Language Models Surpass Neuroscience Experts" shows how these models can synthesize decades of research and predict the outcomes of neuroscientific experiments, often more efficiently than humans themselves. This no longer means competing on the playing field of mere information retrieval; it means surpassing humans in forecasting. Yet this extraordinary efficiency encapsulates a paradox: LLMs’ ability to uncover hidden patterns and correlations unknown to experts highlights new responsibilities in controlling, aligning, and verifying the quality of their predictions.
As AI’s predictive power advances, securing its implementations takes on a critical role. The article "LLMs and Security: MRJ-Agent for a Multi-Round Attack" describes the evolution of threats, showing how multi-round attack agents can bypass sophisticated defenses. We’re no longer talking about simple glitches or temporary weaknesses: the vulnerability landscape is becoming dynamic, with attacks adapting to the defensive responses of the models. In the past, raising a few walls might have sufficed; today, we need a comprehensive defensive strategy, from detecting malicious patterns to calibrating the autonomy of agents, to designing continuous testing. Security thus becomes a fluid process, not a final state reached once and for all.
At the same time, adopting "GenAI in Banking" paves the way for a deep transformation of customer interactions, regulatory compliance, and risk management. Here, the stakes are extremely high: integrating the power of AI into decision-making processes can boost productivity, improve customer experience, and optimize data analysis. However, businesses must contend with cybersecurity challenges, system quality issues, and a gradual adoption process. This is not just a technical question; it’s a strategic consideration that demands investment decisions, public-private partnerships, and ongoing staff training. The goal is not merely to cut costs or increase efficiency, but to build an ecosystem of trust between the financial institution and its stakeholders.
The public sector is also part of this shift. "AI Governance for Public Sector Transformation" emphasizes how the adoption of AI in public administrations requires technical, managerial, and political skills, as well as good governance practices that ensure transparency, reliability, and compliance with regulations. The topic is not confined to technology; it forms an ecosystem of policies, guidelines, staff training, and continuous alignment with human values. This scenario becomes a testing ground for the legitimacy of innovation: if AI in the public sector is not managed with rigor and ethics, there is a risk of undermining citizens’ trust, reducing innovation to nothing more than an empty exercise.
The spectrum of LLM applications grows even wider when considering highly complex and dynamic environments such as gaming. The article "Gaming and Artificial Intelligence. BALROG the New Standard for LLMs and VLMs" shows how testing models in gaming contexts can reveal shortcomings in long-term planning, exploration capabilities, and the management of multimodal inputs. BALROG is a benchmark designed to test the agent-like abilities of the models, bringing to light their limitations in environments that simulate real-world scenarios, where AI must address unpredictable challenges. This approach helps identify weaknesses and gaps in reasoning, driving research toward more robust, versatile models capable of adapting to complex and ever-changing situations.
The need to control and prevent vulnerabilities is no mere add-on. "OWASP Top 10 LLM: Ten Vulnerabilities for LLM-Based Applications" provides a detailed picture of the risks: from prompt injection to the disclosure of sensitive information, from supply chain weaknesses to generated disinformation. Although these vulnerabilities are technical in nature, they raise strategic questions: how can we protect resources, ensure financial resilience, and maintain public trust? Implementing integrated approaches, from data sanitization to defining operational limits and including human supervision for critical actions, is essential.
Companies must invest not only in technical capabilities but also in awareness, internal training, and partnerships with security experts, making security a source of added value.
Taken as a whole, the emerging landscape is one of profound transformation that cannot be left to chance. Companies and institutions are called to integrate expertise, control strategies, and ethical visions. Generative AI is not simply another tool to add to one’s technological arsenal: it is a paradigm shift that forces a rethinking of processes, business models, and governance methodologies. Faced with this scenario, the future belongs to those who can adopt hybrid solutions, balancing the power of LLMs with human oversight, the rigor of security with the flexibility of innovation, the capacity to foresee risks with the determination to seize opportunities.
And as an imaginary ancestor of mine used to say, folding his arms with a smile somewhere between resigned and amused: “You may have the entirety of human knowledge at your fingertips, son, but truly knowing when to stop and look elsewhere always requires a touch of humanity.” And as his words fade, all that remains is the echo of advice no algorithm can ever update with a patch.
Comments